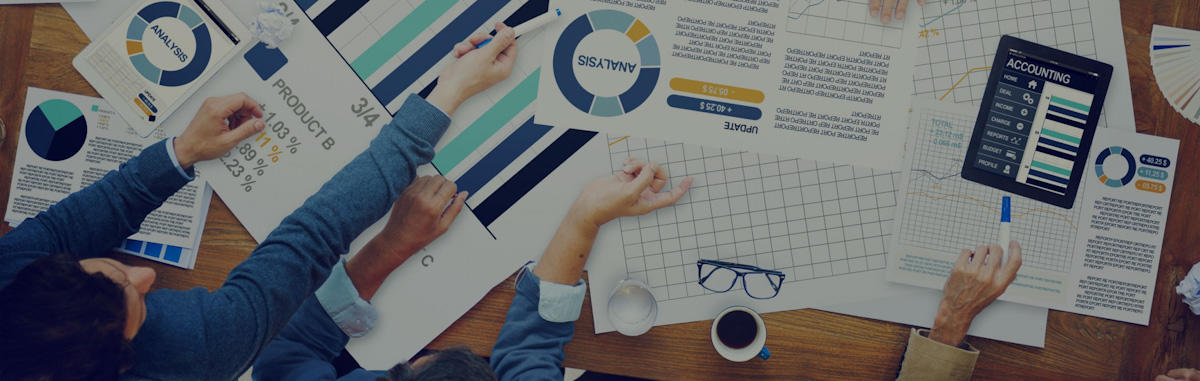
The topic of big data has received a lot of attention in the last several years. The topic is both deep and wide and touches on many other issues, including accounting and data analytics. Let’s discuss what big data is, and how it can be used with accounting data analytics to impact business and industry, as well as the accounting profession.
What is big data?
Big data is the collection of data sets that are so large or complex they cannot be analyzed by traditional databases or tools, such as spreadsheets. Think about the data that might be captured by a car: time-driven, the speed at each time, GPS location, dashboard settings (radio and air), the pressure applied to gas and brake pedals, tire pressure, whether the driver is talking, and the list goes on and on. If this data on your car were collected, it would be difficult for you to analyze. Imagine an automobile maker having to do this for 10 million cars. Each minute of the day delivers a slew of new data. The enormity of the situation is unfathomable. This is big data!
Big data is data that has exploded in one or more of these ways:
- Volume: Often measured in terabytes or more, a typical server’s processing power cannot handle the volume of big data
- Variety: Data from different devices, social media, sensors and sometimes in unstructured formats, like open text
- Velocity: The speed at which the data being analyzed is collected, often in fractions of a second
- Veracity: Inconsistent, incomplete and sometimes inaccurate data
These data typically come in four types:
- Company Data: Product sales, operation metrics, marketing activities, and financial performance
- Consumer Data: Ethnicity, gender, social security numbers, purchases, online behavior, etc.
- Sensor Data: A newer category of data typically about tracking people or things, also known as the internet of things, or IoT
- Syndicated Data: Data acquired from vendors, such as survey data
One of the challenges with data, and even more so with big data is it has to be cleansed, combined, formatted, verified, and secured before it can be analyzed. Oftentimes data is collected across disparate sources and locations and must be linked and merged, or combined before analysis can begin. Security and privacy are two growing challenges. Analytical tools should not include any personally identifiable information (pii), and identity and access restrictions must be in place so that only needed personnel can obtain the data and view the results of the analysis.
With structured data, there are several ways in which it can be collected and stored:
- Categorical: Data that is in categories such as regions of the US, anything yes or no)
- Ordinal: Data that can be ranked, such as excellent, good, average, etc.
- Interval Data: Like ordinal, but the distance between data is meaningful, such as test scores, temperature, or golf handicap
- Ratio Data: All the properties of interval data, but has a natural zero, such as time, height and weight
What is data analytics in accounting?
Big data impacts nearly every aspect of accounting. In auditing, big data can produce more data-driven audits, providing a better experience for the client and the auditor. It can also produce more valuable insights. In advisory services, big data can identify questions, help monitor and improve business performance, and build analytical models that support a variety of product or operational improvements. In tax, big data offers the opportunity to analyze efficiencies more easily, identify tax-related opportunities for improvement, and aid in evaluating global opportunities. Finally, in managerial accounting, big data can help with risk identification and management.
Data analytics for accounting: descriptive vs. predictive vs. prescriptive
Big data is most often used in business to provide descriptive analytics. This includes categorizing and classifying data so that it is useful information. It can help answer questions such as, which region sold the most product?
Taking a step deeper, big data can be used for “predictive analytics”. This enables firms to use historical data to forecast the future. For example, if we spend $1M more on marketing, how will it likely impact sales?
A more sophisticated approach to accounting data analytics involves prescriptive analytics. This practice uses optimization to identify the best alternative or course of action. For example, “what is the ideal spend for marketing?”
Decision models
One of the ways data and analytics are frequently used is with decision models. These are mathematical representations of a problem or business situation used to analyze and make a decision. Decision models typically contain some controlled data, such as the number of salespeople. And, they often include some uncontrolled data, such as interest or unemployment rates. If you were in the mortgage business, a decision model might be used to estimate the ideal number of mortgage processors to employ given the number of salespeople and an estimation of interest rates.
Data visualization
Data visualization is another way in which analytics are used in business and industry. Simple graphs are one example, however, in the last several years a whole field has emerged to help analysts provide more visualization into data and information. Dashboards are a great way to visualize many different metrics quickly, with drill-down capabilities to explore concerns or questions more deeply. A whole category of software specializing in the visualization of big data has emerged alongside the growth of the big data industry.
The topic of big data is sure to continue growing quickly. Professionals and accountants would be wise to continue their professional education in this area.
You can learn more about big data and data analytics for accounting by searching for these topics on the Becker CPE course catalog.